Why solving Agent and RAG Sprawl is essential
Ever wonder what topics are top of mind for our technologists? As part of a series we’re experimenting with, we’re sharing the trends our teams are observing, their insights and approaches. In this edition, Ricky Huynh lead product manager for Walmart's Developer Experience and AI Platform, shares his thoughts on the challenges of Retrieval-Augmented Generation Sprawl (RAG) and Agent Sprawl.
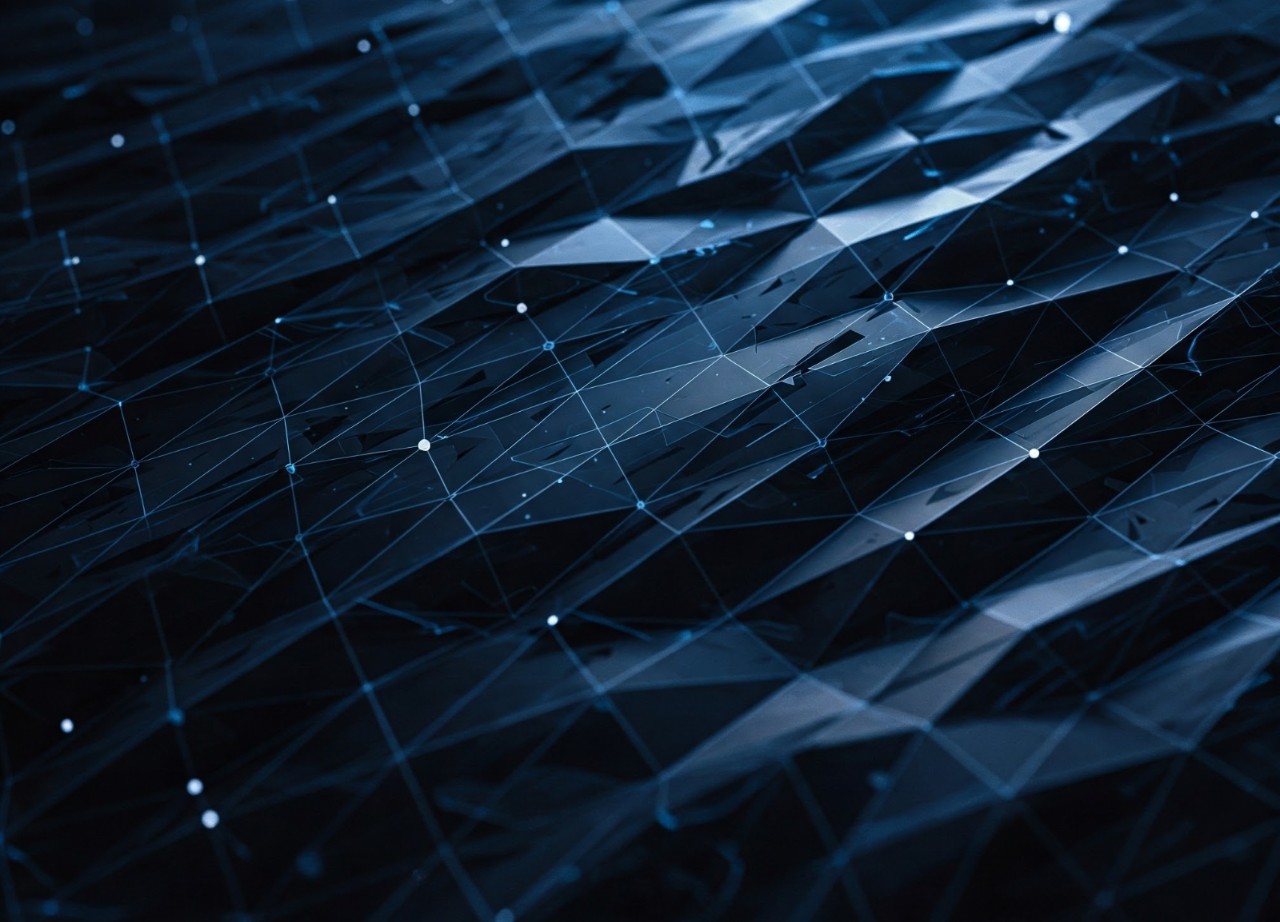
The promise of Agentic AI lies in creating intelligent systems that can autonomously perform tasks, solve complex problems, and collaborate with humans in a seamless manner. However, as we embark on this exciting journey, two critical challenges are emerging: Agent Sprawl and Retrieval-Augmented Generation (RAG) Sprawl.
Understanding these phenomena, their implications, and the necessity for industry standards is paramount to realizing the full potential of Agentic AI, especially within large enterprises like ours.
The impacts isolation and its creation of Agent and RAG sprawl
Agent Sprawl refers to the proliferation of AI agents built in isolation, often with different underlying Large Language Models (LLMs), data frameworks, and code. As various teams or even individual developers create their own AI agents to tackle specific problems, there’s risk of creating islands of agents without a unified communication framework that renders them incapable of interacting, exchanging data, or coordinating efforts with one another. This lack of interoperability hinders the creation of more sophisticated, multi-agent systems capable of tackling complex end-to-end workflows. Just as the early internet struggled before the advent of TCP/IP and DNS enabled seamless connections between computer systems, the “Internet of Agents” envisioned by Cisco’s Head of Outshift Vijoy Pandey requires a similar unifying language.
Further RAG Sprawl arises when different teams independently build their own RAG solutions for their specific AI applications. RAG is a technique that enhances the knowledge of LLMs by grounding them in external data sources. However, without standardization, each team might implement RAG with different methodologies for data vectorization, storage, and retrieval. This leads to inconsistencies, making it difficult for engineers to understand how different RAG systems were built, how to synchronize data across them, and how to ensure quality, security, and extendibility.
Similarities and Differences: Two Sides of the Same Coin
Both Agent and RAG Sprawl stem from a lack of standardization in a rapidly evolving field, and lead to:
- Increased complexity and management overhead: Maintaining numerous disparate systems becomes a significant burden.
- Reduced efficiency and potential for redundancy: Teams might be reinventing the wheel or building overlapping functionalities.
- Challenges in scalability and integration: Connecting and coordinating isolated systems becomes increasingly difficult as their number grows.
Yet, there are key differences in their focus and scope:
- Agent Sprawl primarily concerns the communication and interaction between AI agents, while RAG Sprawl focuses on the creation and management of the data retrieval mechanisms that these agents might use.
- Agent Sprawl deals with the agents themselves as independent entities, whereas RAG Sprawl concerns the underlying infrastructure for providing knowledge to these agents.
These differences matter because they affect how well AI systems work. Agent Sprawl is about how AI agents communicate and work together, while RAG Sprawl is about making sure they have the right information to make good decisions. Both are important for AI systems that can operate independently and intelligently.
The Imperative of Industry Standards
To overcome the limitations imposed by Agent and RAG Sprawl, the development of industry-wide standards is crucial.
The ability for AI agents from different organizations, and even different teams within the same organization, to “freely and seamlessly talk to one another” is a key goal for building an Agentic AI future.
This necessitates a standard communication protocol.
- Model Context Protocol (MCP), announced in November 2024, aims to standardize how models and AI tools connect to data sources. While MCP has garnered interest, it has not yet become a consensus industry standard.
- A collective including Cisco, LangChain, LlamaIndex, Galileo, and Glean has formed AGNTCY, an open-source initiative dedicated to creating an industry-standard agent interoperability language. AGNTCY’s goal is to make it easy for any AI agent to communicate and exchange data with another.
- Agent2Agent (A2A) is an open protocol, designed to enable AI agents from various platforms to communicate with each other and complete workflows. They are partnering with 50 technology companies to establish this as a standard. This protocol is intended to complement MCP, which excels in providing context and tools, while A2A facilitates communication.
Just like how early database implementation led to the standardized language of SQL, we need a standard approach to building RAG Vector DBs. This means setting best practices for handling data, creating vectors, managing metadata, and ensuring security.
While not officially a standard, LangChain and LlamaIndex are two popular options actively shaping best practices for building RAG systems. Their tools and methodologies provide a solid base for building robust and consistent RAG pipelines.
We will also see a trend toward developing vertical AI models based on foundation models and domain-specific knowledge. This concept is akin to having multiple experts handle different pieces of a problem, where one person excels in one area, and another specializes in a different area. This sort of vertical AI model development will become increasingly prevalent, enhancing effectiveness in problem-solving.
Our Journey: Navigating Agent and RAG Sprawl
While our team explores building effective Agentic AI solutions, we are addressing key questions such as which LLMs are best suited for our needs, which embedding models optimize RAG Vector DBs, how to design efficient multi-agent workflows across diverse systems, and the best methods for storing memory within agents to enable seamless handoffs.
As we develop ecosystems of AI agents that automate developer tasks, we must ensure we’re addressing these concerns, while enabling developers to efficiently locate and utilize information spread across multiple platforms and databases. Not having standardization compounds maintenance challenges—the more that’s built, the larger the problem becomes.
One way we’re addressing this is through the establishment of consensus and best practices for managing interconnected systems across orgs. We’re also keeping an eye on trends and readying ourselves to pivot if broader industry standards gain momentum.
Long-term success hinges on continuous iteration, effective communication among AI agent builders, and relentlessly achieving consensus on frameworks for agent and RAG development, while maintaining flexibility to adapt to evolving industry landscapes. We are deepening collaboration with internal teams to share insights and define standards, ensuring a cohesive and scalable Agentic AI ecosystem within Walmart.
Moving Towards a Coherent Agentic Future
The emergence of initiatives and the ongoing evolution of frameworks offer a path forward through the establishment of crucial industry standards. By embracing promising protocols for agent communication and standardizing key frameworks for RAG implementation internally, enterprises can expect more coherent and impactful futures for their Agentic AI endeavors. Addressing these “sprawls” is not just a matter of technical efficiency; it is fundamental to unlocking the true collaborative and problem-solving potential of intelligent AI agents.